4 causes to attend till your staff is extra mature
Many organizations begin their information science journeys by overemphasizing AI analysis and gorging themselves on PhDs of their hiring frenzy. That’s a good suggestion if:
- You’re investing in a analysis division and also you’re not planning to ask your researchers what they’ve carried out for you latterly.
- You already know you’re fixing an issue that’s beneficial, essential, and identified to be inconceivable to resolve with all the present algorithms on the market.
Analysis for analysis’s sake is a high-risk funding and only a few firms can afford it, as a result of getting nothing of worth out of it’s a very actual chance.
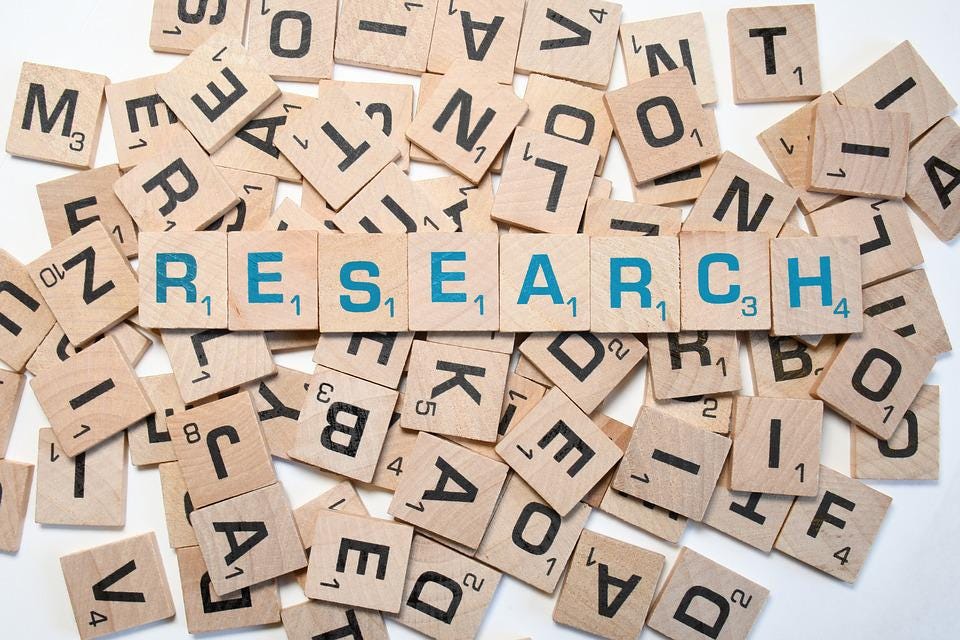
In case your aim is to resolve enterprise issues, listed here are a number of the reason why the AI researcher shouldn’t be your first rent:
- They’re inexperienced persons at your type of information science.
- They’re not skilled with real-world information.
- You don’t have the fitting surroundings for them.
- You haven’t discovered an excellent venture for them.
Learn this paragraph should you’re a researcher: Researchers, I spent a big chunk of my life as one in every of you, so I’ve utmost respect for a way beneficial you will be once you’re directed in the direction of work that respects your abilities. The purpose of this text isn’t to show employers away from you however to keep away from a state of affairs that’s depressing for everybody concerned: being employed into an surroundings that isn’t prepared to understand one of the best of what it’s important to provide. Insist on making employers do their homework to make sure you can flourish in your function.
There are 2 totally different sorts of information science; rent for the one you really need.
- Analysis (e.g. AI analysis, statistics analysis, information science analysis, and many others.)
- Utilized (e.g. AI engineering, utilized statistics, utilized information science, and many others.)
To make use of a kitchen analogy, AI analysis is all about innovating in home equipment — creating blueprints of general-purpose instruments for others to make use of. That’s a really totally different self-discipline from utilized AI, which is extra about innovating in recipes at scale. Utilized AI is the one you want should you’re utilizing information to resolve a enterprise drawback (versus promoting a method for others to resolve their enterprise issues with information).

Each are vastly beneficial and essential, however experience in a single not often interprets to the opposite. Positive, analysis is a good place to search out somebody good and hard-working, however bear in mind that they’ll be studying many of the related abilities for utilized information science on the job. Be taught extra right here.
AI researchers invent general-purpose algorithms and approaches for others to make use of. They’re not right here to resolve your particular drawback utilizing your particular information. The truth is, whereas real-world information could make for a pleasant alternative to indicate their innovations in motion, it doesn’t take a lot for information to get too actual for researchers’ tastes.
Nobody needs the additional strain of contending concurrently with speed-wobbles that come from the answer and from the idiosyncrasies of the info. It’s safer to work with a well known and well-understood dataset that isn’t stuffed with surprises. That’s why AI researchers favor working with artificial datasets and benchmark datasets.

Artificial datasets are simulated (computer-generated from a recipe) to create managed circumstances. AI researchers use them for a similar causes that their labcoat-wearing counterparts want to not conduct their experiments on grubby sidewalks. Like the actual world itself, real-world information will be very messy in ways in which distract from the analysis query.
Researchers want to keep away from real-world information for a similar causes that their labcoat-wearing counterparts want to not conduct their experiments on grubby sidewalks.
The artificial dataset’s conduct and intricacies are a well-known buddy, so debugging is much less nightmarish. Even when the analysis staff strikes to the enterprise drawback’s stay information, they’ll solely do that when they’re certain their dataset performs as anticipated on the artificial dataset, a mindset that isn’t that far off from unit testing in software program engineering.
Benchmark datasets could also be simulated or real-world datasets from previous tasks that the analysis neighborhood makes use of to match outcomes between totally different analysis groups. In case your algorithm scores 65% on one real-world dataset and mine scores 98% on a special dataset, is my algorithm higher than yours? Not possible to say with out an apples-to-apples comparability utilizing benchmark information.
Utilizing benchmark datasets is like everybody agreeing that they’ll strive their microwave prototype out on the identical glass of purified water to see who can get it boiling the quickest. Gross, nobody is planning to really serve that water to any clients.
Which isn’t to say all AI researchers dislike actual world datasets — case research and proofs of ideas are superior, however you’re creating methods that work on the whole, having a sterilized surroundings offers you fewer causes whose results you could battle.
Having a sterilized analysis surroundings offers you fewer causes whose results you could battle.
However I digress. My level is that researchers are unlikely to have a lot expertise working with all kinds of real-world inherited datasets, warts and all, collected with out their involvement. Which is strictly the type of information you’re about to foist on them. Be taught extra about inherited information right here.
Likelihood is that should you’re contemplating making a researcher your first rent, you don’t but have the fitting surroundings to make good use of them. The one factor that PhD packages have in widespread is that they pressure college students to specialize.

Specialists are fantastic and we’d all be at midnight ages with out them, however they’re not skilled to be what you want most in a information science first rent: a ruthlessly pragmatic generalist.
The best information science first rent needs to be a generalist whose ardour is doing no matter it takes to make information helpful. There’s no method that’s too senseless or low-cost if it will get the job carried out.
There are quite a lot of odd jobs that want doing earlier than your staff is able to be productive. All the pieces from organising infrastructure to buying information property to wrangling stakeholders to rising your staff. Your superb rent is somebody who’s able to roll up their sleeves and do no matter wants doing.

To be truthful, many PhD survivors can’t wait to toss their commencement robes onto the bonfire and make a radical profession change, so the diploma itself just isn’t a contraindication for information science. Removed from it. However the need to do analysis clashes with the necessities of being a primary rent within the utilized sphere.
Don’t convey researchers in proper off the bat. It’s higher to attend till your staff is developed sufficient to have found out that what they want a researcher for.
Researchers concentrate on inventing new methods to resolve issues fairly than new options to issues.
Since researchers concentrate on inventing new methods to resolve issues fairly than new options to issues (the excellence is delicate however essential!) you solely want their assist when you’ve verified that the algorithms wanted for fixing your drawback don’t exist but. That’s once you flip to researchers for assist with inventing them.
Wait until you’ve exhausted all of the obtainable instruments earlier than hiring somebody to construct you costly new ones.
Most groups gained’t know upfront whether or not the instruments for fixing their drawback exist already, so it makes extra sense to do issues in the fitting order: earlier than constructing your self that house pen, first verify whether or not a pencil will get the job carried out. Get began first and should you discover that the obtainable off-the-shelf options aren’t providing you with a lot love, then it is best to contemplate hiring researchers.
Researchers solely belong outdoors of a analysis division you probably have acceptable issues for them to resolve — their skillset is creating new algorithms and assessments from scratch the place an off-the-shelf model doesn’t exist — in any other case they’ll expertise a bleak Sisyphean spiral (which might be solely your fault, not theirs). Researchers sometimes spend over a decade in coaching, which deserves at the least the respect of not being put to work on fully irrelevant duties.
Researchers sometimes spend over a decade in coaching, which deserves at the least the respect of not being put to work on fully irrelevant duties.
The appropriate time to rent researchers to an utilized venture tends to be after your analysts helped you establish a beneficial venture and makes an attempt to finish it with utilized information scientists have already failed. That’s once you convey on the skilled inventors.
See my information to the highest 10 roles in information science and AI, organized by hiring order.