Information Science Dysfunctions
Put these information science Venn diagrams away. Sure all 13 of them.
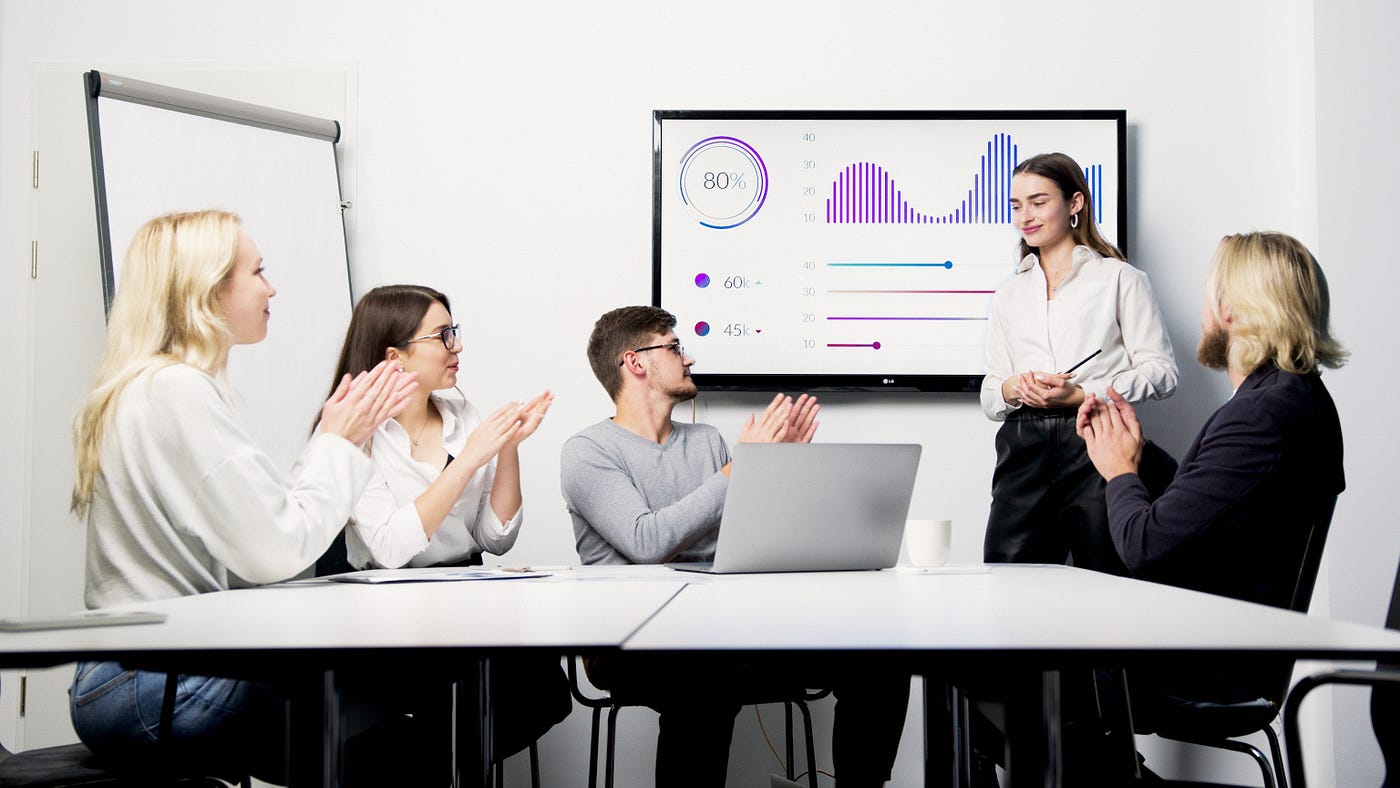
The continued nice resignation that began in 2021, whereas gathering steam and backed by credible information, is nothing new in information science.
At this time is just not about operating explainability on why persons are quitting. (We did this and bravely printed our ideas on how we expect this may be performed with empathy and governance). Somewhat right this moment I need to deal with one key driver of dysfunctional information science groups — administration mistyping what information scientists ought to and mustn’t do.
This explicit dysfunction results in a number of failure modes, together with:
- Information scientists and analysts being pressed into constructing full stack purposes as a substitute of working with software program engineering (the truth is a number of roles in software program engineering).
- Information scientists taking duty for information administration, reporting and constructing dashboards (as a substitute of working with skilled information engineering and enterprise intelligence groups).
- AI researchers spending rising chunks of time deploying and monitoring fashions as soon as initiatives are full (that is nearer to the work of machine studying engineers, and MLOps groups relatively than researchers).
- Information science being conflated with synthetic intelligence (AI strategies can be utilized for insights, AI itself is a distinct self-discipline and has a distinct focus).
This isn’t to say that you’ll want to rent 4 groups to mannequin a desk from a relational database. All information groups begin someplace, and in smaller corporations multitasking is an absolute necessity.
Neither is this saying that information scientists are incapable of doing these duties. A progress mindset is nice, and all roles profit from understanding the work of adjoining groups. However simply because some information scientists can do a job doesn’t imply it ought to be part of core information science duties as a common rule.
Information scientists typically stretch and play a number of roles, doing no matter is critical to carry issues collectively. However this can be a failure mode in itself — as a result of it paradoxically causes administration to suppose issues are working properly so all they want is… extra information scientists. Thus perpetuating the dysfunction.
So, how can we keep away from this?
Higher, exact job titles.
This aligns candidate expectations, saves hiring supervisor time, and may even save your organization being the topic of information science job bait and change threads.
However earlier than we get too far forward, we have to speak in regards to the information science Venn diagram.
Past Information Science Venn Diagrams
When somebody mentions the info science Venn Diagram, an affordable query can be “which one”?
Finally depend there are at the least 13 information science Venn diagrams that are variations of Drew Conway’s authentic. (An enormous thanks to KDNuggets for bringing the checklist collectively.)
I’ve a lot respect to Drew Conway for sharing his concepts — it’s simple to level out how one thing is just not exactly proper for me right this moment whereas forgetting the state of the world earlier than the thought was shared. His preliminary concepts are a terrific landmark to take reference from, and nonetheless applies right this moment.
However the area has matured, and the issue we have to sort out is not only understanding the function of the info scientist, however giving equal consideration to the remainder of the info roles that complement information science. And appreciating that these are established and equal professions on their very own.
Information Science Profession Pathways from the World’s most AI-ready metropolis
A number of years in the past I banded with my fellow crew leads in information and AI to strive tackling this drawback. Serendipitously, what began as a small crew effort ended up pulling us right into a nationwide function definition train in Singapore the place many people had been based mostly. Our work ultimately expanded into defining twelve information, analytics, information science and AI roles.
These are printed within the framework under.
Importantly, the goal was equipping corporations to construct information and AI groups. And as such these roles are HR prepared, and you will see the complete bundle of:
- Function descriptions,
- Taxonomies of technical abilities and mushy abilities,
- Calibrated ranges of every talent for every function, and
- Definitions for every stage of talent.
In the identical interval I used to be inspired to listen to that Singapore’s progress trajectory as an AI hub was supported by successful three awards by the Oliver Wyman Discussion board, Oxford Insights and the Worldwide Improvement Analysis Centre, and the IMD that at the moment put it because the world’s most AI-ready metropolis. (As a knowledge scientist I’m perennially curious in regards to the interior workings of rankings, so I dug a bit deeper and located by way of Stanford HAI’s world AI vibrancy instrument that Singapore’s success was doubtless calculated on a per-capita foundation.)
No matter awards, the framework is virtually helpful and now represents one in every of the hottest sources for profession pathways for information and AI professionals.
A Expertise Framework for Information and AI Careers

There are Twelve Distinct Roles in Information and AI
These distinct roles are listed under. They’re break up into 5 function ‘households’, and the y axis roughly denotes seniority throughout the household:
- Information Analyst/Affiliate Information Engineer
- Enterprise Intelligence Supervisor
- Enterprise Intelligence Director
- Information Engineer
- Senior Information Engineer
- Information Architect
- Synthetic Intelligence/Machine Studying Engineer
- Senior Synthetic Intelligence/Machine Studying Engineer
- Information Scientist/Synthetic Intelligence Scientist
- Synthetic Intelligence Utilized Researcher
- Head of Information Science and Synthetic Intelligence
- Chief Information Officer/Chief Synthetic Intelligence Officer
Somewhat than repeat the content material on the location, a extra helpful framing could be to take a lens of somebody new to information and AI careers.
We wished to assist new entrants to information, analytics and AI reply the query: which information function would I finest thrive in?
And to reply this query, I suggest 4 particular cuts of the info:

Enterprise Intelligence is a longtime area with its personal profession path.
Enterprise intelligence (“BI”) is a longtime area with many overlaps with information science. It contains figuring out enterprise wants, making ready and analysing information and presenting insights. Nonetheless, these alone doesn’t imply it ought to be subsumed into information science.
BI requires a mixture of abilities which are concurrently near the shopper, near the info, and near the product or platform of alternative. These abilities embody information analytics, information engineering, information governance, information visualization and stakeholder engagement – with full checklist accessible through the abilities framework web site right here.
Equally vital is what’s lacking — whereas development in BI imply that many fashionable BI instruments now supply some predictive functionality, duty for modelling is just not on their required talent set.
A very fascinating trait can be how each BI crew we polled constructed their follow round a small variety of business BI instruments.
BI is its personal distinct profession pathway and it could come as a shock to some information scientists that even now BI professionals and jobs typically outnumber information science ones.
The query that gives essentially the most info on a job is commonly about whether or not you produce insights or merchandise.
If I used to be interviewing for a job and will solely ask one query to get info on a knowledge science function, I’d not ask whether or not the job requires deep studying, operates in a devops surroundings, or requires information of causality.
The only query that gives me essentially the most info of your information science function is: “is your output primarily insights or merchandise?”
Or requested in a barely completely different approach, is the output of your function primarily consumed by people, or by machines?
To the extent the reply is ‘insights’, you drift in the direction of the best of the framework , and can doubtless be extra ‘scientist’ than ‘engineer’. That is the place you double down on understanding your enterprise drawback area, require cautious inference abilities, and channel communication abilities to assist your stakeholders to do the identical. You’d additionally profit from sitting as shut as you possibly can to the choice makers you are attempting to assist.
Conversely to the extent the reply is ‘merchandise’, you drift in the direction of the left and in the direction of the ‘engineer’ persona. You could be ‘nearer to IT’, profit enormously from an understanding of utility improvement and integration, and can doubtless function in agile product or supply groups.
There are particular roles the place PhDs are necessary. However they’re comparatively few and much between.
The third query is one that usually comes up once I converse to graduates – whether or not one requires a PhD to be a knowledge scientist. In recent times, this has shifted barely to ‘would having a PhD assist my profession’.
Whereas the specifics of your state of affairs is an important, it’s value mentioning that there’s a particular set of roles that profit enormously from a PhD. These are sometimes in machine studying or a particular subfield of synthetic intelligence (akin to picture processing or NLP).
These fall beneath the AI Utilized Researcher function, and are sometimes marked by the R&D nature of their work, publishing papers as a part of their work scope, and skill to provide patentable AI options.
Entry stage candidates ought to be conscious that analyst roles have a number of meanings.
If you’re simply switching into information and your supply letter says ‘information analyst’, that may be an unhelpful time period(!).
It is because the ‘information analyst’ function is used a number of completely different job households, and your expertise shall be considerably completely different relying on which household the function sits in.
One interpretation of a knowledge analyst is the function that analyses information for insights, and the opposite is the function that analyses information for engineering.
To additional complicate issues, each use the time period modelling. However when a knowledge scientist mentions modelling, it typically implies becoming curves to distributions and constructing representations of the info for a predictive or inference job. However when a knowledge engineer mentions modelling, this use is related to information constructions and schemas to retailer, handle and carry out operations on information.
Whichever variation you end up in, know who you might be and I belief you’ll develop into a mannequin worker (sorry).
Closing ideas: Constructing Your Greatest Staff!
Going from information scientist to supervisor, director and past requires new roadmaps for the brand new personas and roles in your expanded groups. We did this so that you don’t must, so be happy to leverage and adapt this framework to your wants. And please don’t hesitate to achieve out when you have questions, suggestions, or simply want a sounding board in constructing out your crew.
Now if you’re a supervisor and also you simply employed an econometrician to ‘construct your information cloth so you are able to do predictive analytics on prime’, or employed a ‘BI developer’ to optimize your advertising and marketing spend as a result of ‘you need the outcomes on a dashboard’, you might have considered trying learn this text once more. However go take a stroll in a random forest first.