A information for knowledge scientists evaluating options
Monitoring is vital to the success of machine studying fashions deployed in manufacturing methods. As a result of ML fashions should not static items of code however, quite, dynamic predictors which rely upon knowledge, hyperparameters, analysis metrics, and plenty of different variables, it is important to have perception into the coaching, validation, deployment, and inference processes in an effort to forestall mannequin drift and predictive stasis, and a bunch of further points. Nonetheless, not all monitoring options are created equal. On this publish, I spotlight three must-haves for machine studying monitoring, which hopefully serve you nicely whether or not you might be deciding to construct or purchase an answer.

First, fashions must be evaluated within the context of the enterprise operate they intention to serve. Such operate is commonly realized a number of steps downstream from the mannequin. Exterior of the lab, many AI-driven purposes contain a number of fashions working in tandem. Moreover, the conduct of the fashions will possible rely upon knowledge transformations that are a number of steps upstream. Thus, a monitoring answer which focuses on single mannequin conduct is not going to seize the holistic image of mannequin efficiency because it pertains to the worldwide enterprise context and can fail to uncover lots of the points that start or finish exterior of the mannequin. A correct evaluation of ML mannequin viability solely comes from full course of visibility — having perception into your entire dataflow, metadata, context, and overarching enterprise processes on which the modeling is based.
For instance, as a part of a credit score approval software, a financial institution could deploy a collection of fashions which assess credit score worthiness, display for potential fraud, and dynamically allocate trending affords and promos. A easy monitoring system may be capable of consider any considered one of these fashions individually, however fixing the general enterprise drawback calls for an understanding of the interlocution between them. Whereas they could have divergent modeling objectives, every of those fashions rests upon a shared basis of coaching knowledge, context, and enterprise metadata. Thus, an efficient monitoring answer will take all of those disparate items into consideration and generate unified insights which harness this shared info. These may embody figuring out area of interest and underutilized buyer segments within the coaching knowledge distribution, flagging potential cases of idea and knowledge drift, understanding the combination mannequin impression on enterprise KPIs, and extra.
The most effective monitoring options are capable of prolong to all course of levels, together with those which don’t contain a mannequin element.
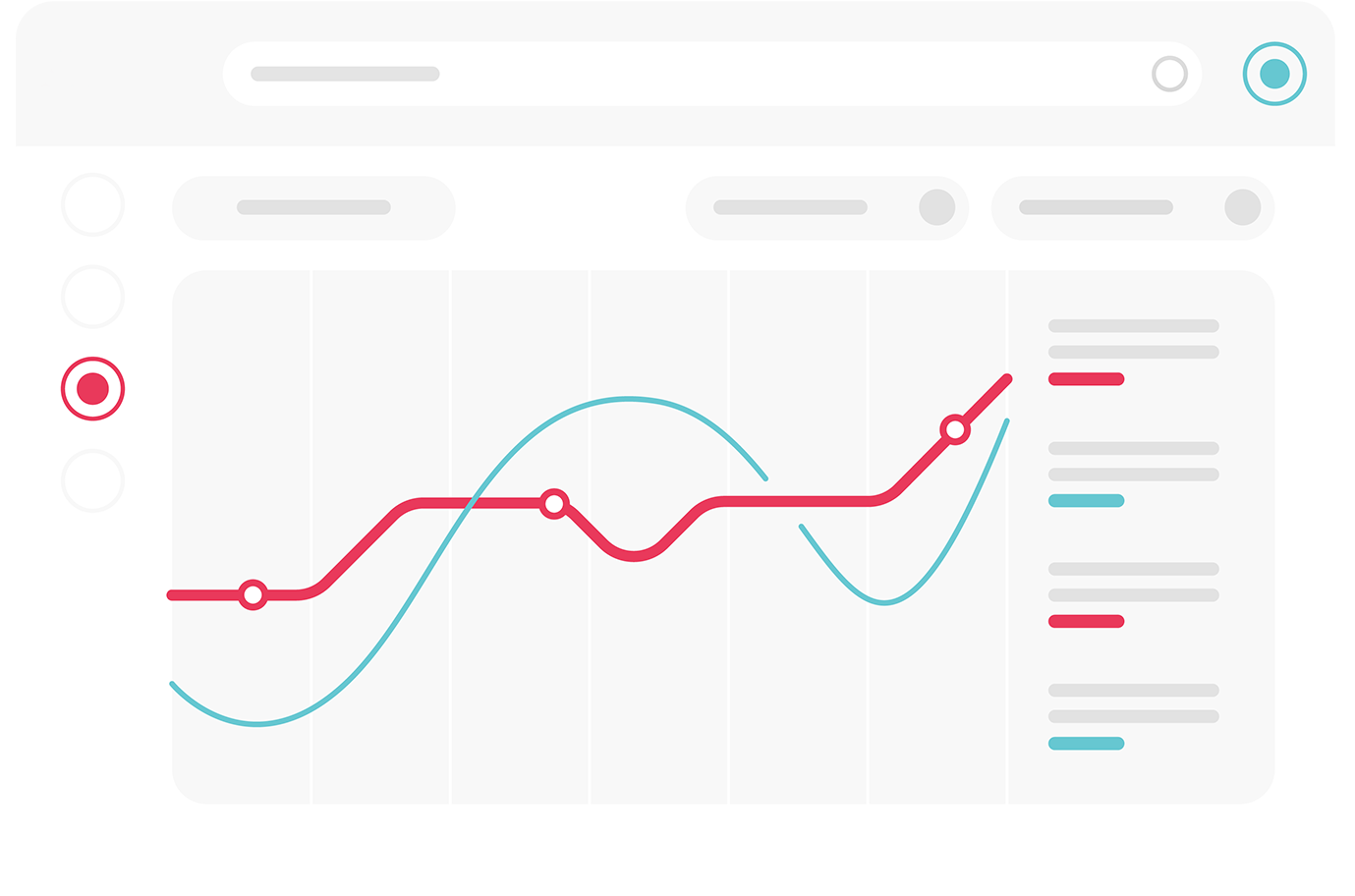
A standard false impression is {that a} monitoring answer ought to merely allow visualization and troubleshooting of the widespread metrics related to an ML mannequin in manufacturing. Whereas that is useful, visualization and troubleshooting implies that you’re already in “investigation mode”. Worse but, you could be “fire-fighting” after the enterprise complained {that a} KPI dropped (and requested “what’s unsuitable with the mannequin?”).
So, how about being extra proactive?
How about detecting points weeks and even longer earlier than total efficiency declined?
You must count on your monitoring answer to mechanically detect issues when they’re nonetheless small, in granular segments of knowledge. Permitting you ample time to take corrective or preemptive motion. The which means of “mechanically” deserves some additional elaboration right here. Some monitoring instruments will present dashboards that will let you manually examine subsegments of knowledge to see what’s performing nicely and what’s not. Nonetheless, this type of facile introspection requires painstaking guide intervention and misses the higher level, which is {that a} true monitoring answer will be capable of intrinsically detect anomalies through its personal mechanisms with out exterior reliance on a person to offer a speculation of their very own.
The extra granular you get, the extra it is best to take note of noise discount. It’s anticipated that single anomalies would propagate points in a number of locations. It’s through detection of the basis causes of points that the monitoring really succeeds, not simply by flagging surface-level knowledge discrepancies or the like.

Completely different ML methods have completely different knowledge and stream, completely different enterprise cycles, completely different success indicators, and various kinds of fashions. You must significantly doubt “plug-and-play” monitoring options.
An entire ML monitoring answer needs to be configurable to any drawback and throughout all of its elements. It ought to be capable of absorb any mannequin metric, any unstructured log, and, actually, any piece of tabular knowledge and make it simple to:
- Assemble and repeatedly replace a single efficiency database
- Create and customise dynamic visualizations and reviews
- Setup and tweak computerized, granular insights and notifications
One easy instance for the necessity for configurability lies within the distinction between methods in which you’ll achieve (close to) actual time suggestions for mannequin constancy (e.g., shopper advice methods) and ones during which a suggestions loop requires human intervention and extra time (e.g., fraud detection, credit score scoring and extra).
Most enterprise ML groups are engaged on a wide range of ML tasks on very completely different enterprise issues. Consequently, monitoring necessities are broad and require nuance and adaptability to accommodate the variations. If you’re on a kind of groups, you might have established sturdy knowledge science requirements, a unified stack for knowledge preparation, mannequin growth and deployment. Now, will you be capable of monitor and govern your methods with unified requirements and a single answer? You must undoubtedly count on to take action.
Given the ever-increasing hype round machine studying, there exist many options which is able to take an ML mannequin and supply superficial insights into its characteristic conduct, output distributions and fundamental efficiency metrics. Nonetheless, options which exhibit full course of visibility, proactive, clever insights, and complete configurability are a lot, a lot rarer. But, it’s these three attributes that are key for squeezing the best efficiency and downstream enterprise impression out of ML fashions. Subsequently, it’s essential to guage any monitoring answer by means of the lens of those three must-haves and be certain that it supplies not solely mannequin visibility however a extra international and full understanding of the enterprise context.