The frontier of deep studying methods in pc imaginative and prescient
What’s presently happening within the pc imaginative and prescient neighborhood? In case you are an avid pc imaginative and prescient fanatic like me, listed here are a number of of my favorite papers in 2021–2022 that I consider may have huge affect going into 2022.
Disclaimer: These papers are what I consider as “foundational” papers. There are quite a few papers extending past them with nice insights, and I’ll steadily add the hyperlinks right here for reference.

In case you are following deep studying methods for some time, you’ve got in all probability heard of BERT, a self-supervised approach utilized in languages the place you masks out a part of sentences for pretraining for the betterment of studying implicit representations. Nevertheless, the identical approach wasn’t transferrable onto CNNs in studying higher characteristic extractions — not till not too long ago when imaginative and prescient transformers ViTs are launched.
With a transformer structure, He et al. investigated whether or not masking turns into relevant, and the outcomes are motivating: a masked autoencoder accompanying ViTs are proven to be an efficient pretraining approach for downstream duties corresponding to classifications and reconstructions.
Paper Hyperlink: https://arxiv.org/abs/2006.11239
Associated Papers:

Then again, whereas ViTs have proven to be superior in quite a few papers for imaginative and prescient duties, one work stood out in analysing the basics of convolutional networks (ConvNet). Liu et al. centered on “modernising” a convolutional community, bringing in components which are confirmed empirically helpful to ViTs, and confirmed {that a} ConvNet can certainly obtain outcomes just like that of ViTs on giant datasets like ImageNet.
Paper Hyperlink: https://arxiv.org/abs/2201.03545
Associated Papers:
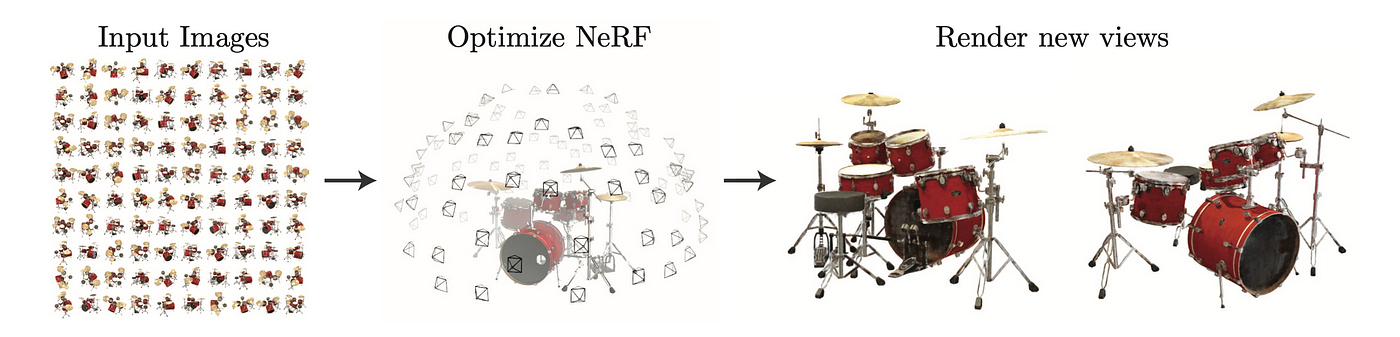
Shifting to the three-dimensional area, there are additionally astonishing breakthroughs in reconstruction and novel view synthesis. Mildenhall et al. proposed to make use of a fully-connected community to characterize a 3D form. That’s, given some coordinates and viewing angle, the community outputs the corresponding color of the actual area. This enables new photographs/views of the item at unknown angles to be generated in excessive and correct qualities.
Paper Hyperlink: https://arxiv.org/abs/2201.03545
Associated Papers:

When discussing generative networks, one typically take into consideration GANs or VAEs. Surprisingly, diffusion fashions are the excelling household of fashions lately. In easy phrases, a diffusion mannequin steadily provides noises to a picture throughout its ahead course of, whereas studying a reverse course of concurrently. Thus, a reverse course of turns into a generative mannequin the place a picture could be “extracted” out of pure random noise just like the info distribution the mannequin is skilled in.
Paper Hyperlink: https://arxiv.org/abs/2006.11239
Associated Papers:
Thanks for making it this far 🙏! I might be posting extra on totally different areas of pc imaginative and prescient/deep studying, so be a part of and subscribe in case you are to know extra!