Contemplating the “Invisible Hole” between Measurements and their Which means
In an period of empiricism, the place insights which can be “data-driven” are robotically deemed superior, quantification is significant. Certainly, the measurement of constructs and phenomena is on the core of empirical science, analysis, and reasoning. Nevertheless, this quantification is commonly difficult, and is interrogated accordingly in the course of the analysis course of. Nonetheless, the standards for “good” quantification are arguably agreed upon; These objectives are the minimization of bias and variance.
Throughout a go to to HEC Paris, Nobel laureate Daniel Kahneman spoke of the results of each each bias, evoked closely in Considering, Quick and Gradual, in addition to noise, which has been the topic of his newer writing. Throughout his speak, I raised questions on whether or not the concentrate on the aforementioned dichotomy could trigger us to miss sure flaws in empirical fashions. With the luxurious of additional reflection and more room to articulate these ideas, I wish to elaborate on my considerations on this article.
Bias and variance: A brief however crucial overview
To mannequin a phenomenon, empirical analysis frameworks depend on varied approaches to measuring a assemble inside the inhabitants. For example, one could wish to measure the hazard to well being posed by coronavirus. To realize this, one may acquire loss of life charges (i.e., samples) from varied hospitals , and common them to realize our true purpose of estimating the loss of life charge within the general inhabitants. One would then hope that this strategy to estimating the general loss of life charge has low bias, and low variance, and that bias and variance lower because the variety of observations will increase. Nevertheless, the determine beneath illustrates the important thing points that would come up throughout this estimation course of.

One situation could also be that the estimator has excessive variance (top-right), that means that pattern hospitals differ considerably within the loss of life charges they report, regardless of being dispersed across the “true” inhabitants loss of life charge. Such a end result would point out that the estimation of the true loss of life charge are scattered, loosely-speaking. One other situation could be if the estimation course of is biased (bottom-left), that means that it persistently skews estimations in a sure path. Maybe, for instance, our strategy concerned sampling hospitals in poor areas, which could exhibit increased loss of life charges on account of inferior medical assets. Combining these two points, an estimator could also be each biased and exhibit excessive variance (bottom-right).
Motivated by our tacit crucial to reduce each bias and variance, maybe probably the most generally used mannequin in empirical analysis is peculiar least squares regression (a.ok.a. OLS). Depicted beneath, this strategy primarily takes in information, and finds a development line that minimizes the whole error between the road and information factors it represents (i.e., the “sum of squared errors”). In line with the Gauss-Markov Theorem, the OLS strategy is BLUE — i.e., the most effective linear unbiased strategy to modeling such phenomena, that means that it’s the strategy that yields minimal bias and variance. Constructing extra subtle and non-linear fashions, empirical researchers take into account the bias-variance tradeoff, once more with the purpose of minimizing these values. Therefore, the collection of an empirical modelling strategy is guided closely by the minimization of bias and variance.
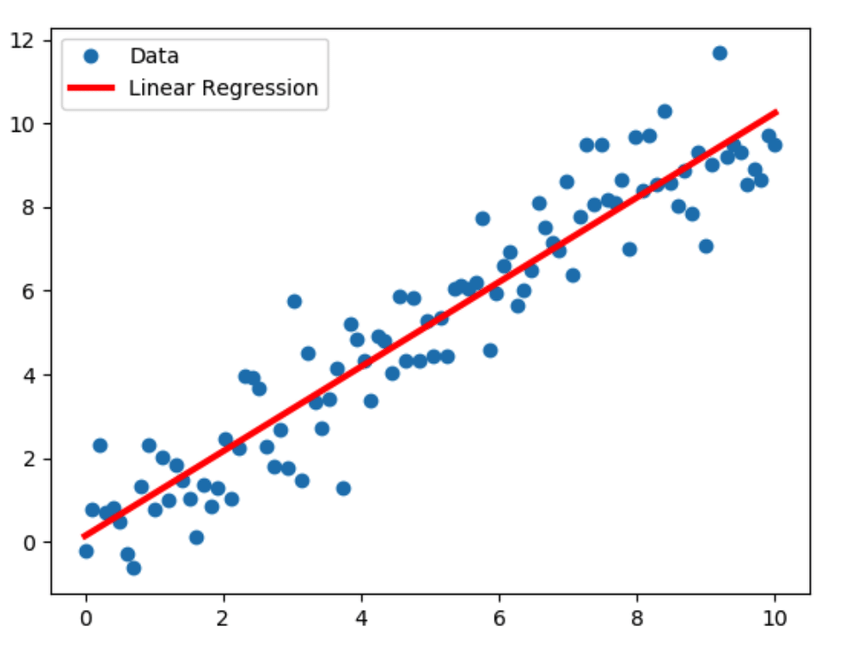
My argument could be that, being blinded by the minimization of bias and variance, we frequently miss a extra elementary query: what’s the underlying goal? What are these factors on the regression chart above? In spite of everything, discussions of both of those elements are much less related if the goal is poorly outlined. Usually, we don’t dig deep sufficient into this goal, however fairly settle for the metric that almost all simply affords quantification.
Is there ever a “true” goal?
Within the instance of coronavirus loss of life charges, allow us to take into account the context by which we is likely to be desirous about such a metric. On this explicit case, it’s probably that the science behind this estimation could be supposed to tell choices or insurance policies. For instance, a loss of life charge could affect particular person choices on vaccination, or insurance policies on lockdowns. A mannequin that focuses on loss of life charge as an final result would therefore provide prescriptions to optimize (on this case, reduce) this pre-determined metric.
Even with an correct (i.e., low bias, low variance) estimate of loss of life charge, this metric is just one of quite a few elements one might have thought of within the broader context of minimizing hurt or maximizing basic well being. Thus, loss of life charge is a proxy for an underlying assemble of curiosity, comparable to basic welfare. Even with the hole between “true” loss of life charge and measured loss of life charge being minimal, there can stay an invisible hole between our chosen metric and the true goal for which this metric is a proxy.

By failing to contemplate the space between a metric used and the true assemble of curiosity, we run the chance of being seduced by the simplicity of quantification. For example, one could seem rigorous by citing a low loss of life charge as an final result of curiosity and proposing insurance policies accordingly (e.g., eradicate lockdowns). Certainly, this was an argument utilized by libertarian voices, who typically appear to model themselves because the “cheap” and “goal” ones, as in contrast with others comparable to liberals. Nevertheless, to counter such an argument, one needn’t depend on questioning the accuracy of the measurement; Somewhat, a greater technique is likely to be to query the selection of metric itself.
Given the potential lack of data ensuing from one’s alternative of metric, one may ask: can a quantifiable measurement be the “true” goal? This query is analogous to the favored query: “Is every little thing quantifiable?”. Certainly, on the one hand, one can assemble a metric to affiliate with something; Nevertheless, in doing so, one inevitably loses nuance in representing the extra summary assemble that they really want to characterize. Even in instances the place an thought is cleanly measurable (e.g., weight, inhabitants), very hardly ever are we truly desirous about such a simplistic assemble as such. Somewhat, it’s extra probably we use a measure like weight as a illustration for a extra summary and multi-dimensional idea like “well being”.
Transferring past bias and variance
Whereas the main target of empirical science has been in establishing correct measurement scales, analysis also can profit from reflecting upon the underlying assemble of alternative, in addition to the selection of metric as a proxy. Ignoring such questions would give the phantasm that the scientific course of is grounded in goal measurements. Nevertheless, the alternative of measures as a proxy for the true underlying assemble could be very typically subjective, and could be manipulated to serve a particular agenda (e.g., elimination of lockdowns primarily based on low loss of life charges).
Despite these points, there could also be events by which the lack of nuance related to quantification is appropriate. For example, once I publish a paper the place the phenomenon of curiosity is the consumption of on-line movies, one may ask questions comparable to: Did they view your complete video? How a lot consideration had been they paying to this video? One could make related analogies in observe, as an illustration if a agency is monitoring “visits” to its web site. Whereas quantifying such constructs utilizing a easy tally could lose nuance, some data loss is important to assemble empirical fashions, that are abstractions that may information our actions and choices.
Past the world of science, we are able to enhance the way in which we body our fashions in widespread parlance. Even informal statements we hear in day by day life comparable to “I’m biased” must be examined — what precisely are you biased in the direction of or in opposition to? What’s the true assemble you are attempting to judge, and why do you assume that the method by which you arrived at your choice is distorted? If the difficulty itself is inherently subjective (e.g., I’m biased as a result of I’m a fan of the Paris Saint-Germain soccer membership), then the phrase “biased” is misused as a result of there is no such thing as a “true” goal being estimated, no matter measurement challenges. Alternatively, if the bias is in the direction of a choice that one arrives at systematically (e.g., As a supporter of Emmanuel Macron, I’m biased), then one ought to ask what’s stopping this individual from conducting their evaluation otherwise.
With the rise of quantification and favoritism in the direction of “goal” analyses, we run the chance of ignoring philosophical points such because the hole between measures and their theoretical targets. Whereas even discussing this hole requires us to enter a messier realm of nuanced subjectivity, it’s higher to do that explicitly than sweep the mess beneath the rug implicitly. It will be helpful for scientists to contemplate creating pointers round this observe to in the end make their empirical analyses extra convincing.