How explainable machine studying might be utilized for sample recognition in breast most cancers analysis
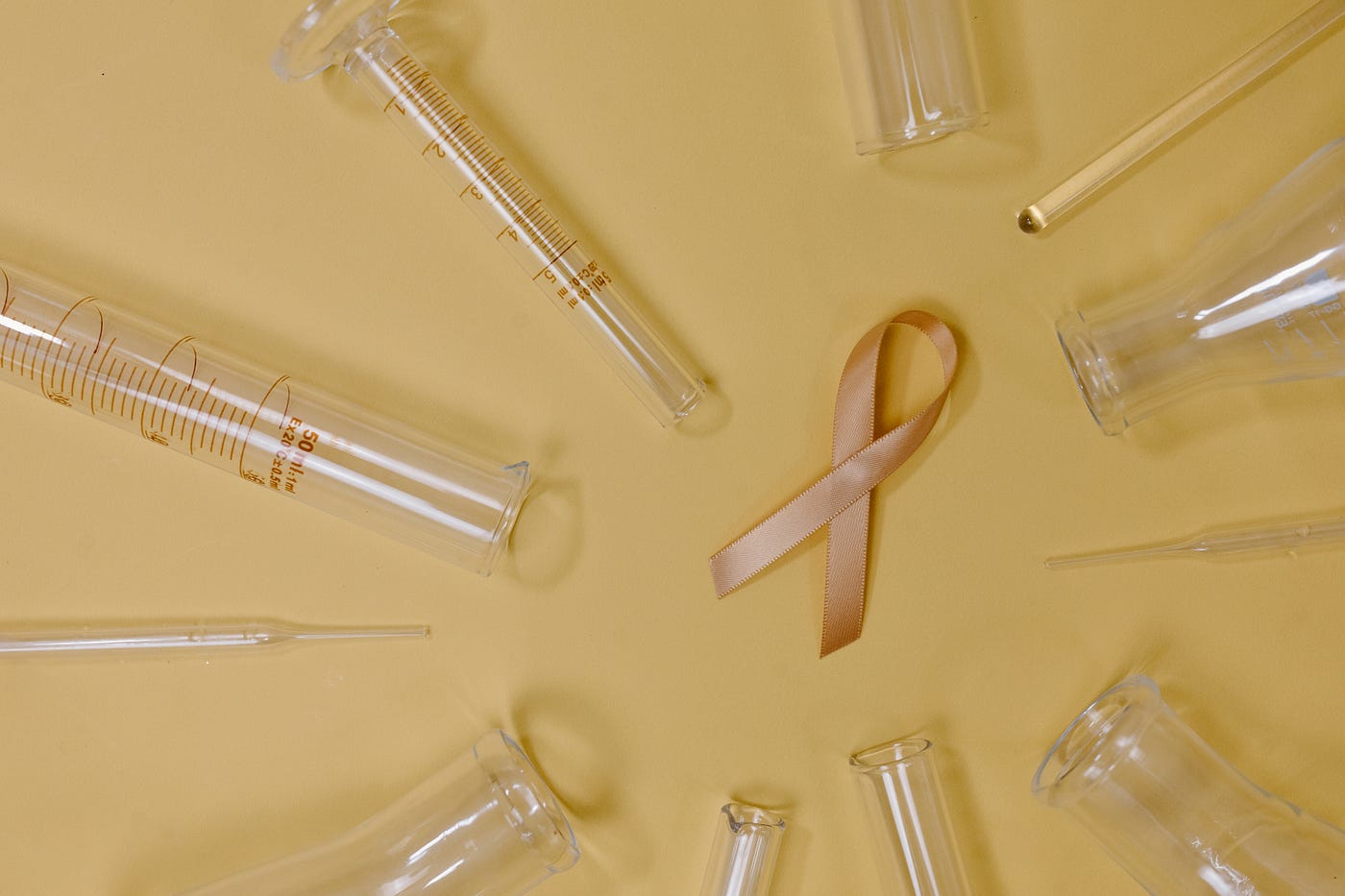
Over the previous few years, there was a number of hype round how machine studying will rework many industries. One business talked about regularly is well being care. Within the following weblog put up, we’ll discover a kind of impression that may be made. We are going to do that by inspecting an article that was printed within the journal Nature in 2021. The article’s title is Morphological and molecular breast most cancers profiling by explainable machine studying.
This put up can have three sections: Function of the examine, Strategies / Outcomes of the examine, and Why this issues for Oncology and well being care.
Function
Traditionally, there was a scarcity of integration between varied profiling methods inside oncology analysis. That leaves some disconnect concerning molecular information and morphological information for most cancers properties. The researchers on this examine got down to create a hyperlink by machine studying that bridges the hole between these two varieties of profiling methods. If profitable, this method may help with speculation era for connections between cell varieties and molecular properties.
Strategies / Outcomes
Machine Studying Algorithm
The machine studying methodology that the examine used was based mostly on a method referred to as layer-wise relevance propagation (LRP). Layer-wise relevance propagation is a kind of explainable machine studying algorithm. As a substitute of solely giving an output for a selected enter, the method can spotlight a very powerful enter options used for the output given. For conditions utilizing photographs, the options which can be highlighted are pixels. The result’s a heatmap over the enter picture displaying which pixels had the very best impression on swaying the output. Pixels with excessive coloration depth correlate to excessive relevance scores for pixels given the classification. The fundamental equation for this course of might be denoted as:
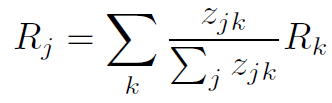
j and okay characterize neurons at two consecutive layers within the neural community. Zjk represents how a lot neuron j contributed to creating neuron okay related. The R is the relevance scores of j and okay.
The LRP computes attributes that designate the whole contribution of an enter function somewhat than the sensitivity to an enter variation that you’d get in attention-heatmaps. For a extra technical rationalization of how layer-wise relevance propagation works, please discuss with the next paper: Layer-Smart Relevance Propagation An Overview.
Utility of the Algorithm
Now that we mentioned the overview of how layer-wise relevance propagation works, we’ll discover how the analysis staff was ready to make use of this with most cancers morphological and molecular breast most cancers information.
The staff first created a picture database (Berlin Most cancers Picture Base, B-CIB) with annotated patches of microscopy picture information. Utilizing LRP, the staff was capable of distinguish most cancers cells from tumor-infiltrating lymphocytes (TiLs). TiLs are lymphocytic cells that may infiltrate tumor tissue and acknowledge and kill most cancers cells. The density of their presence can be utilized as a function for the prediction of affected person survival. LRP permits for a visible illustration of their density.
The researchers then predicted molecular options utilizing morphological picture information as enter for the algorithm. Basically, making an attempt to derive insights into the molecular properties of a affected person by scanning a picture. The information used for coaching consisted of mixing picture and molecular profiling information. Given the intent of this a part of the examine and the data out there by the dataset for coaching, handbook spatial annotation was not required. They may have the algorithm establish patterns inside the photographs by feeding it the molecular information and morphological information throughout coaching. To scale back the dimensionality of the classification activity, they used a excessive vs. low classification method for the totally different molecular options. The algorithm gave a classification based mostly on a prediction of whether or not a molecular function’s expression was excessive or low, given a morphological picture as enter. Two genes that scored excessive for ranges of expression have been CDH1 and TP53.
Significance of CDH1 AND TP53 genes
The algorithm producing a excessive expression rating for these molecular options is sensible based mostly on a priori data of the impression they’ve involving breast most cancers.
The TP53 gene is named a tumor suppressor gene. It regulates the extent of mitosis in a cell. When this gene is mutated extreme cell division happens and tumors are fashioned.
Mutations of CDH1 have been linked to most cancers development by rising proliferation, which is a rise in cell numbers, and metastasis the place most cancers growths are developed away from the fatherland. The protein produced by CDH1 is E-cadherin which is essential for cell adhesion, which retains cells collectively, and it’s thought {that a} genetic change on this gene can result in most cancers cells having the ability to detach from the first website of the tumor extra simply, resulting in metastasis.
The staff additionally experimented with predicting the spatial localization of molecular options. This prediction exhibits spatial areas statistically related to sure molecular function expressions. This info can be utilized to create hypotheses about how related particular parts of the tumor microenvironment are for the presence of molecular tumor profile options—driving the invention of potential hyperlinks that will exist between the histological options of the tumor proven within the picture and the molecular options which may be expressed. These hyperlinks can result in candidate lists being made out there for molecular options. These lists would include molecular options associated to breast most cancers.
In the end, the machine studying method revealed spatial and morphological options which can be statistically related to expressions of assorted molecular options. This info was displayed by a heatmap that visualizes how most often, molecular options have particular associations with the morphological teams examined for (most cancers cells, TiLs, and stroma (help cells)).
To check the validity of the outcomes, the staff used a method referred to as immunohistochemical (IHC) staining to check the outcomes with the heatmaps generated from the machine studying algorithm. They utilized a quadrat take a look at to indicate the associations between the 2. A quadrat take a look at can be utilized to measure spatial randomness for some extent sample. The quadrant take a look at measures spatial randomness through the use of a chi-squared take a look at. Total, the IHC patterns mirrored the patterns predicted from the machine studying algorithm validating the machine studying method.
Why
The examine we checked out at the moment is a superb instance of how machine studying can be utilized for most cancers analysis functions. It won’t essentially exchange instruments already in place, however it will possibly act complementary to them. Some use instances for oncology analysis relate to speculation era by new patterns that will emerge from a machine learning-based method. Examples of the hypotheses that might be generated from the examine explored on this article would principally be associated to new relationships being found between non-spatial molecular options and spatial info. These relationships may help for extra refined tumor grading and new candidate lists for potential focused therapies.
In an business the place sample recognition is essential, machine studying can act as a guiding mild for pushing discovery additional.