The work of knowledge science groups will be intertwined with cloud and different tech property, which might make them a part of budgetary questions raised about cloud spending. That is simply one of many methods knowledge scientists have expanded past some outdated expectations of the work they do and the property they leverage. If steps should not taken to kind out how such assets are used, organizations may see knowledge science contribute extra to prices reasonably than returns.
Shane Quinlan, director of product administration with Kion, spoke with InformationWeek about how knowledge science has advanced, and methods knowledge scientists can effectively use the cloud.
Are knowledge scientists working exterior the field in contrast with what has been anticipated of them? What totally different angles are they taking to satisfy their duties?
Information science wasn’t one thing actually on my radar once I began working in know-how. The thrill began in 2015-2018, when knowledge science grow to be the factor. New positions began getting created and we began getting issues like DataOps and MLOps. Huge data–if you slap that onto any firm, then gold mine.
I acquired pulled into it round that very same timeframe, shifting from a job the place I used to be working, principally supporting federal and regulation enforcement prospects, leaping into healthcare. Switching from net and endpoint options to analytics. That was my first leap into knowledge science.
Now I’m seeing it from a distinct angle as a result of our product focus is far more on platform and infrastructure administration. I’m it from the cloud in direction of knowledge science as a substitute of from knowledge science in direction of the cloud.
What are the influences and elements that have an effect on the approaches that knowledge scientists take? As knowledge scientists leverage the cloud, what do they should be extra aware of?
I see two traits. One is round adjustments in know-how and availability. Early on, it was sort of the Wild West. There have been tons of recent service choices, know-how stacks, and the skillsets have been actually divergent and began to be slightly bit extra accessible.
Information science was this huge world. You had every little thing out of your Excel knowledge scientist actually utilizing Microsoft Excel, to an expectation that you can write Java functions that might carry out knowledge features and supply totally different output. You had mathematicians, you had statisticians, you had software program builders, and also you had people who had extra of a enterprise intelligence-analyst position all coming on the identical area and looking for alternative ways to satisfy their expectations.
That’s once you noticed a push for higher person interfaces, making the event aspect much less of a requirement. That’s the place you might have the introduction of notebooks like Jupyter and Zeppelin and derivations thereof to make that slightly bit simpler. You had like a human interpretable code and not-code interface with the way in which that you just’re shaping knowledge. Behind the scenes, I believe there’s been this large explosion of the way to form that as nicely. You’ve gotten tech like DBT that’s making the information transformations loads simpler. Applied sciences that have been centered across the Apache Hadoop ecosystem have now shifted and morphed and moved in all places making it much more moveable. Apache Spark will be run in all types of various contexts now.
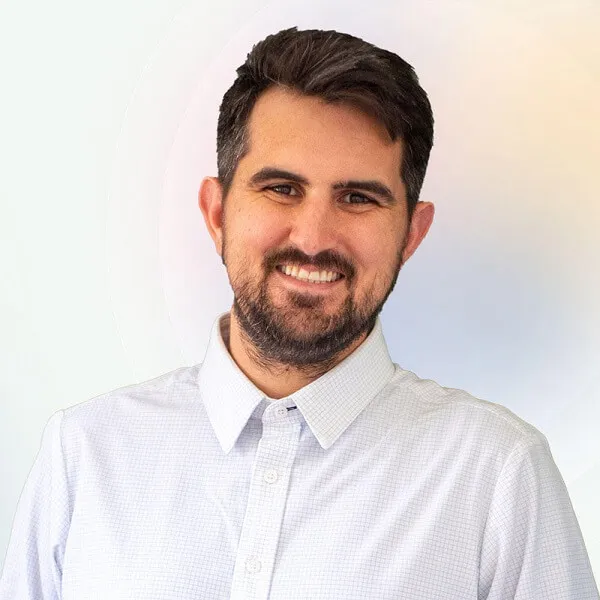
There’s been a drive in direction of a extra user-centric mannequin of knowledge science. Extra user-friendly, extra person interfaces, extra simply interpretable. You’ll be able to carry widespread skillsets like Excel or BI instruments or SQL and do sufficient with that to make a distinction.
The opposite aspect of that may be a development-centric method, the place as a developer it makes knowledge science extra approachable versus asking mathematicians to study to be builders.
One other piece is that this stress round bigness and simply how a lot knowledge is required to create the sorts of insights you must present enterprise worth. The CEO of Touchdown AI [Andrew Ng] has made this large push for ‘huge datasets are dumb’. [Big datasets are] losing cash, they’re losing time. Cleaner, smaller datasets are literally extra impactful. [Ng has said you don’t always need “big data,” but rather “good data.”] You see this stress between the standard method of ‘get all the information and study as a lot as you’ll be able to from it,’ versus cleaner, smaller cheaper, extra environment friendly datasets offering that perception.
A few of it comes again to people attempting to do magic with what they’d. Method too many I’ve talked to have been like, “We now have all this knowledge; we have to do one thing with it.”
Okay. Nice. What?
And so they’d say, “Effectively, we have to run some machine studying so we are able to see what we are able to discover out.”
It doesn’t work that means. You need to carry an precise scientific mindset to know what speculation you’re testing through the use of these fashions. It requires a really particular mindset to have that a lot self-discipline and the way in which you method problem-solving and worth creation by way of knowledge science strategies versus, ‘I’ve knowledge; please do issues.’
When IT budgets come underneath scrutiny with knowledge scientists making use of the cloud, what will be accomplished to kind out their group’s wants?
The wonderful thing about cloud is you utilize it once you want it. Clearly, you pay for utilizing it once you want it however typically occasions knowledge science functions, particularly ones you’re working over giant datasets, aren’t working constantly or don’t should be structured in a means that they run constantly. Subsequently, you’re speaking a couple of very concentrated quantity of spend for a really quick period of time. Shopping for {hardware} to do this means your {hardware} sits idle except you’re very energetic about ensuring you’re being very environment friendly within the utilization of that useful resource over time.
One of many greatest benefits of cloud is that it runs and scales as you want it to. So even a tiny can run a large computation and run it when they should and never constantly.
That provides challenges, in fact. “I fired this factor off on Friday, I come again in on Monday and it’s nonetheless working, and I by chance spent $6,000 this weekend. Oops.” That occurs on a regular basis and a lot of that is determining set up guardrails.
Typically knowledge science will get handled like, “You recognize, they’re going to do no matter they should.”
Within the growth world, we’ve began to have language to talk to this risk-taking, experimental, ‘don’t punish failure, we study from failure’. We’ve been in a position to carry that language in, however we’ve ignored knowledge science.
Are there some greatest practices for balancing and managing the improvements knowledge scientists may wish to reap the benefits of?
In case your knowledge science division is younger and small, cloud-first sounds scary however will set you up for achievement down the road. If you wish to make these decisions on {hardware} investments, then you may make them on the applicable time as a substitute of considering you must purchase {hardware} upfront after which go to cloud later, which is infinitely more durable.
Guardrails don’t need to be rocket science. They are often easy. Easy will be very efficient.
What to Learn Subsequent:
An Insider’s Have a look at Intuit’s AI and Information Science Operation
To Resolve Your Information Science Expertise Hole, Embrace Variety