Understanding correlation gained’t assist you to with choice making and initiatives measurement in a enterprise setting. A strong grasp of causality is what you want.
Whether or not you’re a Information Scientist coping with Determination Science, Advertising and marketing, Buyer Science, or efficient A/B testing, Causal Reasoning is a high ability you must grasp in your profession. Disentangling cause-effect relationships is usually ignored in enterprise and is a largely poorly understood apply. Many key selections are taken on anectodical proof, many fallacious conclusions are pushed by spurious correlations. This could go from spamming prospects for no motive to disastrous waste of cash as a result of incorrect initiatives.
Fact is that mastering causality when coping with human behaviour or socio-economic methods, by definition chaotic and multivariate methods, is rattling exhausting. But having the ability to body issues in a causal vogue drastically helps give some order to the chaos.
Let’s begin with a use case
A quite common use case will assist make issues clear:
Your media firm has a subscription-based income stream. You supply month-to-month and yearly plans, the standard stuff. Drawback is that in the previous few months you’ve gotten been experiencing a big drop in renewals. All people is in a frenzy about it, so your advertising supervisor rushes to supply your prospects a 20% low cost for renewal to all the buyer base, with out choosing a management group (so no Randomized Management Trial).
After the marketing campaign you take a look at a random buyer and guess what, they renewed after taking the low cost. However right here comes the issue, how are you aware that they renewed as an impact of your marketing campaign? What in case your random buyer would have renewed their subscription whatever the low cost? You might actually go and ask, however typically that is unimaginable. What can also be unimaginable is to decide the counterfactual actuality of your single random buyer, which is observing what would have occurred to their plan had you not despatched them a reduction.
You might be dealing with the Basic Drawback of Causal Inference, which is which you could solely observe one final result at a time for every particular person.
We face this drawback each time we make an intervention that doesn’t have a deterministic final result. An intervention is one thing that we are able to manipulate to attempt to get a distinct causal final result. For instance, deciding to supply a reduction is an intervention, since we are able to additionally determine the alternative. On the flipside, gender or ethnicity may be causal results however don’t qualify as interventions, since we can not change any person’s gender.
Within the determine beneath we characterize this case graphically. Within the blue contour what we are able to observe. You supplied a reduction to all of our prospects, so you may observe two potential outcomes: Y=1 or Y=0. The orange space is the counterfactual world, what would have occurred had you not supplied the low cost. It’s a digital world you can’t observe instantly however on which you’ll be able to postulate inference.
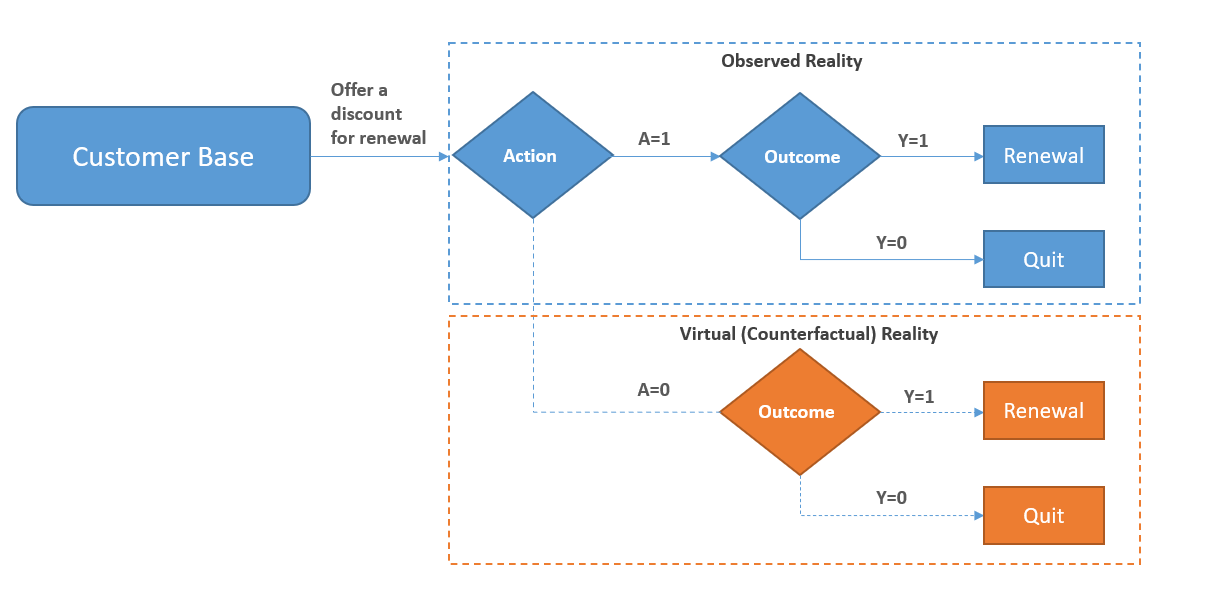
At this level we are able to add a little bit of notation that can assist additional down:

The Motion, which any more we are going to consult with because the Therapy, can take two doable values, and so does the result. We observe an final result after giving a therapy, the counterfactual is what we might have noticed had we not given it. Subsequently we’ve got a causal impact solely the place:

Clearly, in case your random buyer renewed their membership each within the noticed world and within the digital world, the low cost was not essentially what precipitated the renewal (the impact).
Estimating the Causal Impact
Estimating the causal impact is the job of scrutinizing the unobservable parallel actuality. Or no less than making an educating estimate of what occurs in there. It may be framed as discovering the distinction between two anticipated values:

That is the distinction between the typical final result for all our prospects in the actual world and the typical final result within the digital world, the parallel actuality the place no person acquired a reduction for renewing. Solely by estimating Y⁰, you do have some probability of accurately measuring the influence of the marketing campaign.
Causality vs Conditioning, the unending confusion…
At this level, you would possibly begin questioning why we’re bothering an excessive amount of about all of this cause-effect philosophical stuff. After some digging, you discover out that not all prospects had been supplied a reduction, however solely those that had given express consent to contact. In spite of everything, you may need a management group to measure the marketing campaign’s effectiveness, which makes your finance supervisor very comfortable.
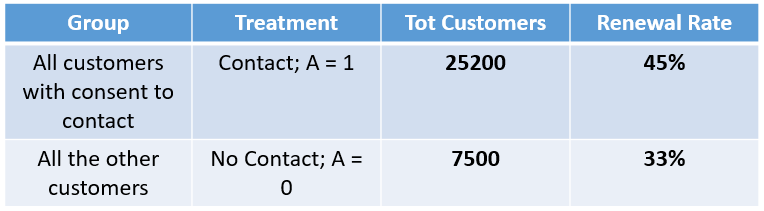
The leads to fig 5. are very encouraging, the marketing campaign was successful with 13 pp distinction between the 2 teams. Nonetheless, your finance supervisor nonetheless finds one thing fishy about it. As a matter of truth, we have to estimate what a inhabitants would have accomplished in a parallel dimension if handled in another way than what occurred in actuality, we’re not making an attempt to check the subpopulation of consumers who had been handled versus the subpopulation of untreated prospects. Put it in math notation:

The common therapy impact is just not the identical because the distinction in final result between the subpopulation of the handled vs the subpopulation of the non handled.
In essence, as per determine 7. we’re occupied with inferring what would have occurred within the digital actuality for each the subpopulation. We would want to estimate:
- The doubtless final result for the purchasers contacted had they not been contacted. That is the Common Therapy Impact on the Handled (ATT) we outlined above.
- The doubtless final result for the client not contacted had they been contacted.
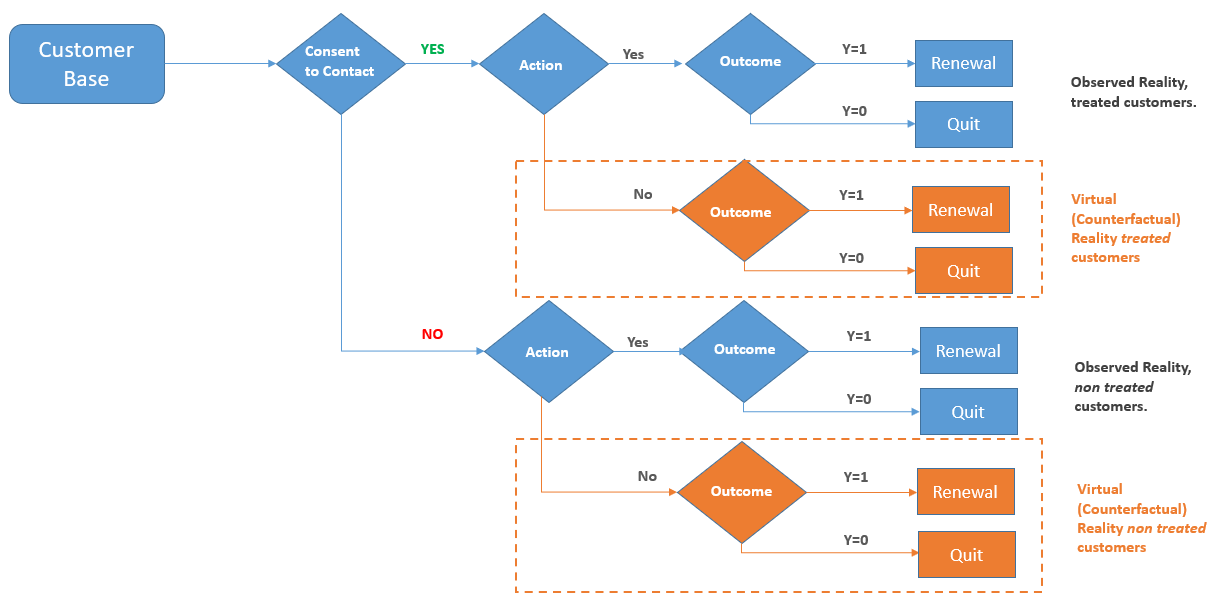
Evaluating the 2 subpopulations is just not incorrect typically. It will be tremendous if we created a Randomized Management Trial from an preliminary inhabitants and a totally random cut up between the 2 teams. However on this case, who will get the therapy (the motion) depends upon offering consent to contact. However who offers the consent to contact for business initiatives? Most likely individuals who don’t care about privateness? Or individuals who intend to be extra engaged together with your product or web site?
Breaking the Ignorability Assumption
After analyzing issues additional you discover that individuals who joined the loyalty program are extra doubtless to offer consent to contact and to be concerned in a renewal marketing campaign. However guess what, these individuals are additionally extra more likely to spontaneously renew no matter your campaigns.
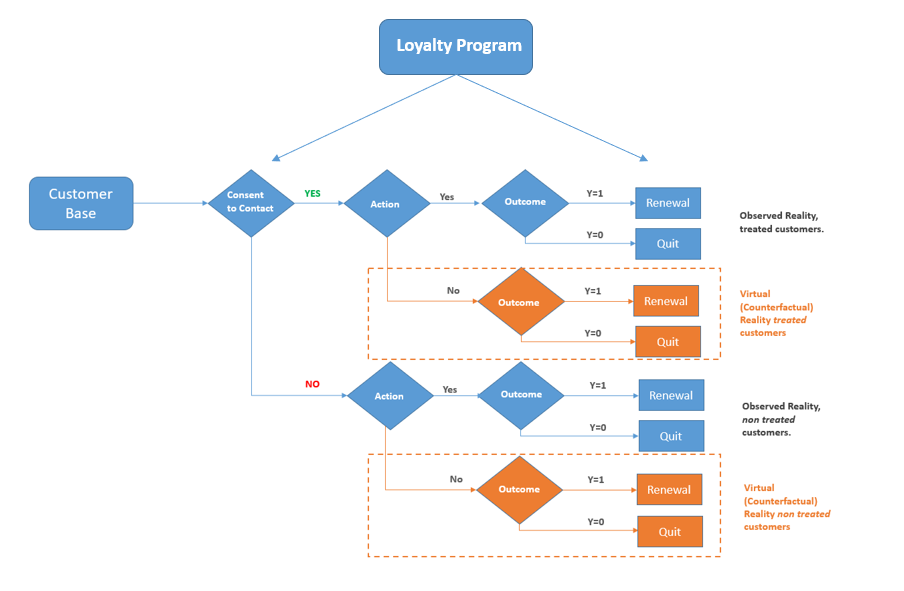
The consent to contact causes your prospects to be within the marketing campaign, which in flip causally impacts the renewal chance. The loyalty program subscription each impacts the consent and the renewal. At this level, we can not ignore how every buyer ended up within the marketing campaign, therefore we can not assume that the therapy project was one way or the other unbiased of the potential final result.
As such the loyalty program is a confounder, which is a variable that causes you to be unable of determining whether or not the noticed outcomes had been as a result of your advertising prowess or to some backdoor results of the loyalty program. To clear the confusion, it is advisable management the confounder by stratifying for this variable.
Let’s increase the marketing campaign final result by including a variable X for loyalty program subscriptions. Once we add covariates to the teams the state of affairs begins altering.

We are able to estimate the causal impact of the marketing campaign by evaluating the goal and the management group alongside totally different strata. For many who joined the loyalty program, the marketing campaign was simply barely efficient, for the others the marketing campaign had no influence or was barely detrimental. At this level, we are able to measure the common therapy impact of the marketing campaign by taking a weighted common of the consequences noticed at every stratum as proven in fig.9
Figuring out and controlling for the fitting confounders is a step ahead towards wanting into the digital actuality of counterfactuals. On this instance, you don’t understand how your marketing campaign’s prospects would have behaved with out the marketing campaign, however you may make an informed guess by prospects much like them who weren’t within the marketing campaign. On this case, the loyalty program subscribers who didn’t present consent to contact (even when in actuality you could wish to stratify for a lot of extra doable confounders).
The result’s that your marketing campaign was much less profitable than initially anticipated, presumably even producing extra prices in giveaway reductions than advantages. Higher not inform it to your finance supervisor.
References:
- I realized many of the concepts and the notation from the fantastic course on Causality by Jason Roy : https://www.coursera.org/be taught/crash-course-in-causality
- One other elementary reference level is The Guide of Why: The New Science of Trigger and Impact, by Judea Pearl and Dana MacKenzie, Penguin, 2019.