Rice College engineers have devised a mechanism for folks to help robots in “seeing” their environment and finishing up duties.
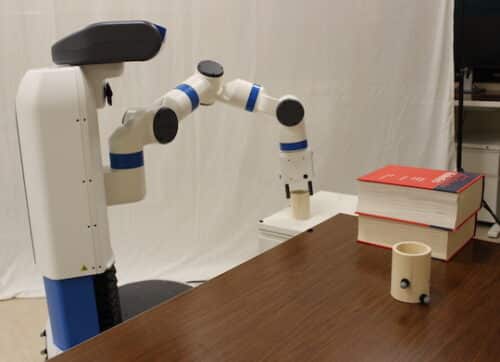
The Bayesian Studying IN the Darkish (BLIND) method is a novel answer to the long-standing problem of movement planning for robots that work in environments the place all the things shouldn’t be at all times clearly obvious. In response to the report, the algorithm constructed by the researchers retains a human within the loop to “improve robotic notion and, extra crucially, stop the execution of unsafe movement.”
To take action, they built-in Bayesian inverse reinforcement studying (during which a system learns from constantly up to date data and expertise) with well-established movement planning approaches to assist robots with “excessive levels of freedom,” or many shifting elements. The Rice lab used a Fetch robotic, a seven-jointed articulated arm, to know a small cylinder from one desk and carry it to a different, nevertheless it needed to undergo a barrier to do it.
When obstructions obscure a machine’s “view” of its vacation spot, the its programmers should be exact concerning the motion of every joint at every level in its trajectory. BLIND inserts a human mid-process to switch the choreographic prospects — or greatest guesses — supplied by the robotic’s algorithm, somewhat than setting a trajectory up entrance.
“BLIND permits us to take data within the human’s head and compute our trajectories on this high-degree-of-freedom area,” Quintero-Peña mentioned. “We use a particular manner of suggestions known as critique, principally a binary type of suggestions the place the human is given labels on items of the trajectory,” he mentioned. These labels seem as a sequence of interconnected inexperienced dots that symbolise completely different paths. The human accepts or rejects every motion as BLIND strikes from dot to dot, refining the trail and avoiding obstructions as successfully as attainable.
“It’s a simple interface for folks to make use of, as a result of we will say, ‘I like this’ or ‘I don’t like that,’ and the robotic makes use of this data to plan,” Chamzas mentioned. As soon as rewarded with an accredited set of actions, the robotic can perform its process, he mentioned. “Some of the necessary issues right here is that human preferences are laborious to explain with a mathematical method,” Quintero-Peña mentioned. “Our work simplifies human-robot relationships by incorporating human preferences. That’s how I believe purposes will get essentially the most profit from this work.”
Click on right here to view their demo on YouTube.